The Future of Prompt Engineering - Part 3
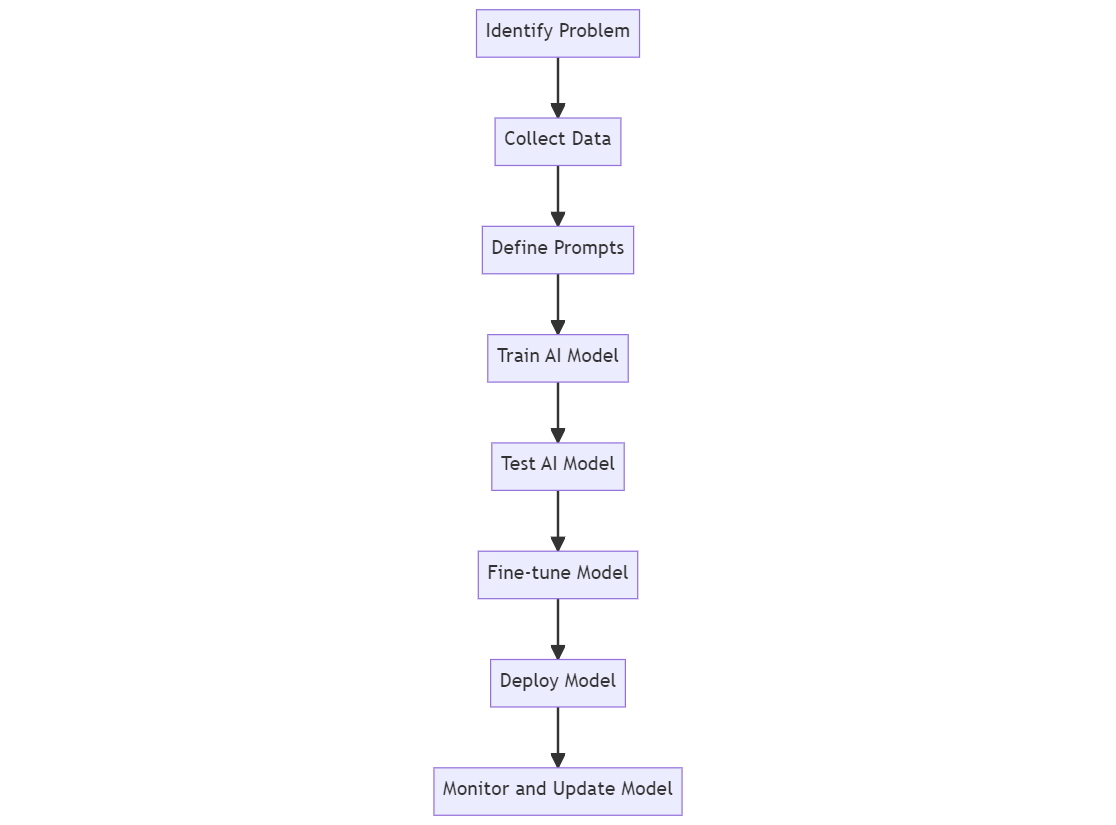
Welcome to the final part of our three-part series on the dynamic world of Prompt Engineering. In Part 1, we laid the foundation by exploring the fundamentals, while Part 2 dived into advanced techniques and real-world applications. In this instalment, we will peer into the future of prompt engineering, examining emerging trends, potential challenges, and the exciting possibilities that lie ahead. Join us as we explore the dynamic world of prompt engineering and its continuous evolution!
Emerging Trends in Prompt Engineering
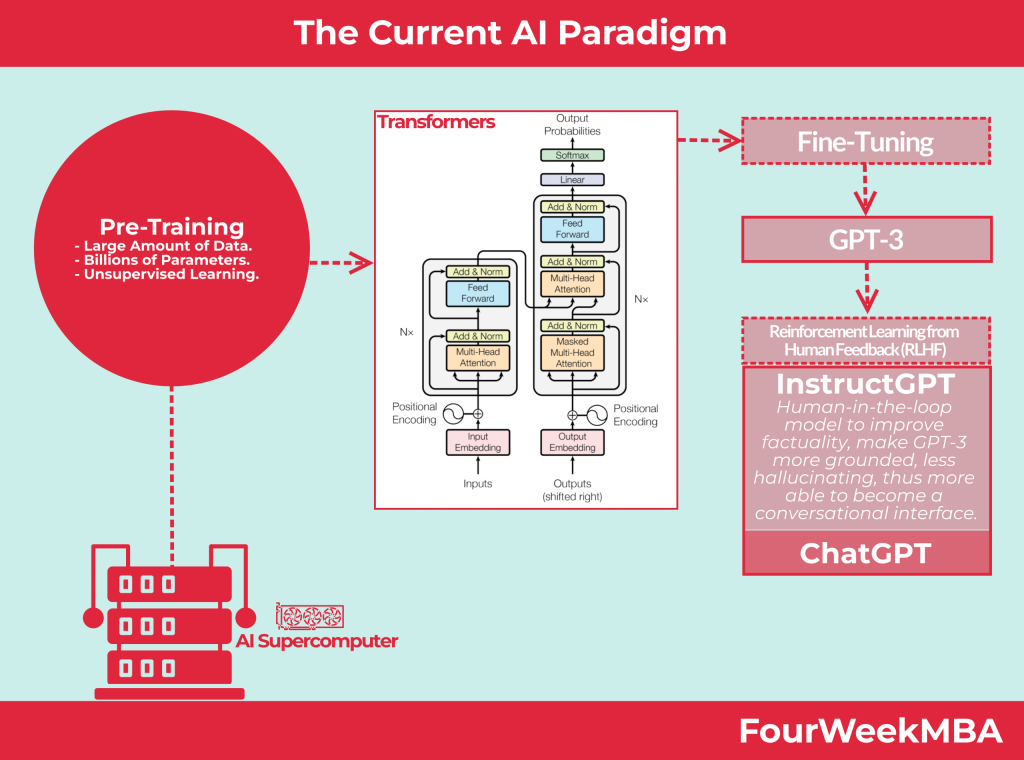

As technology progresses, prompt engineering continues to evolve. Let's explore some of the emerging trends that are shaping the future of this field:
Adaptive Prompting: Adaptive prompting involves dynamically adjusting prompts based on user feedback and preferences. AI models can learn from user interactions, adapt to their needs, and generate responses that align more accurately with individual preferences. This personalised approach enhances the user experience and fosters a deeper level of engagement.
OpenAI's Prompting Tools: OpenAI and other organisations are actively developing and refining tools designed explicitly for prompt engineering. These tools aim to simplify the prompt engineering process, making it more accessible to a broader range of users. Such advancements will empower individuals and organisations to harness the power of AI with greater ease and efficiency.
Collaboration and Collective Intelligence: Prompt engineering can benefit from collaboration and collective intelligence. Communities of prompt designers and researchers are forming and sharing insights, best practices, and prompt templates. This collaborative approach fosters innovation, accelerates learning, and pushes the boundaries of what is achievable in prompt engineering.
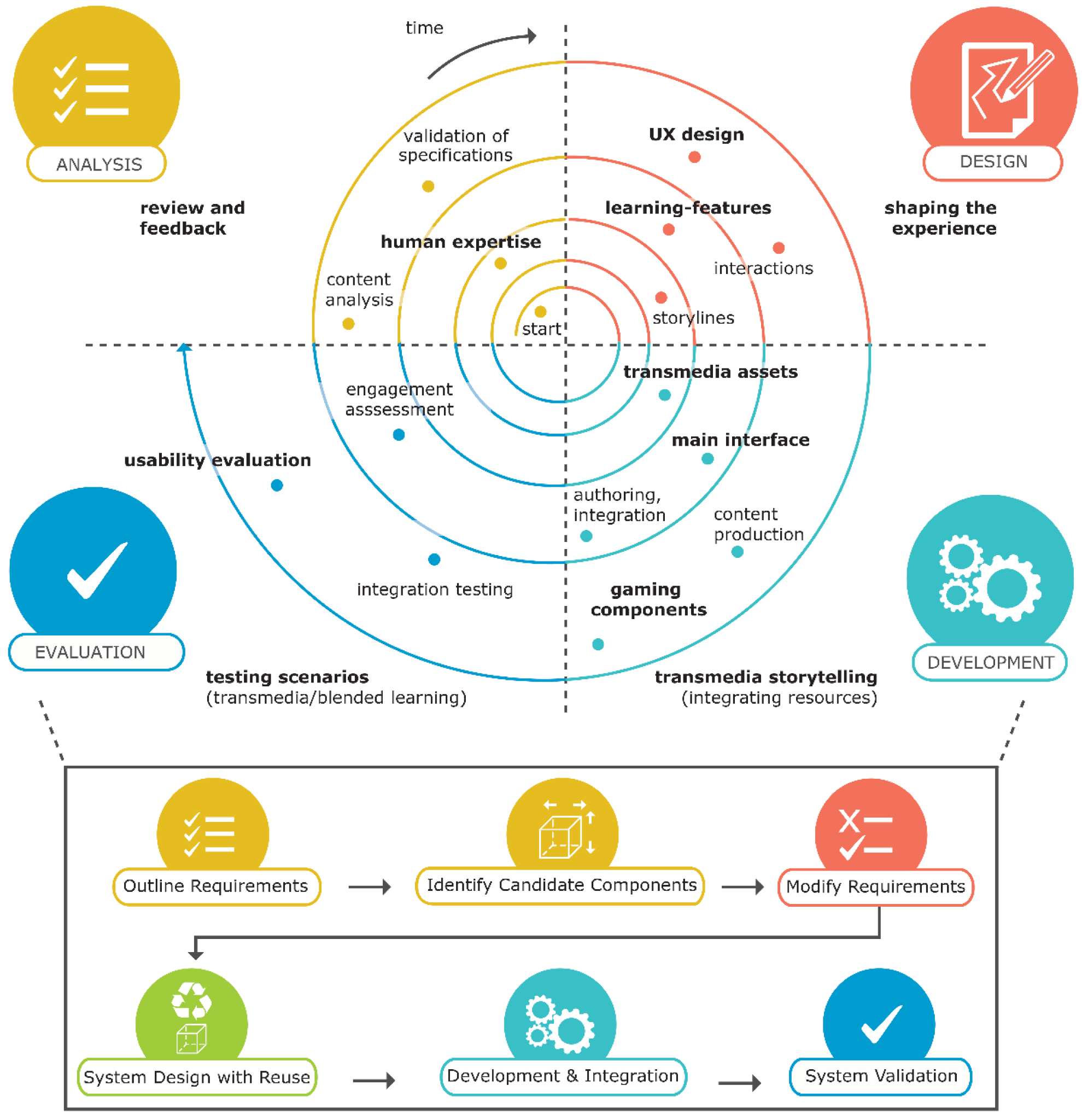
Human-in-the-Loop Approach: The human-in-the-loop approach involves integrating human feedback and expertise into the prompt engineering process. By incorporating human insights and judgments, AI models can be guided to produce more reliable and contextually appropriate responses. This collaborative approach not only improves the accuracy and relevance of AI-generated outputs but also promotes human oversight and control.
Continual Learning and Adaptation: Prompt engineering is moving towards a continual learning and adaptation paradigm. AI models can be designed to learn from user interactions, update their knowledge, and refine their responses over time. This iterative process allows prompt engineering systems to improve their performance, adapt to changing user needs, and stay up-to-date with evolving trends and information.
Domain-Specific Prompt Engineering: As prompt engineering becomes more specialized, there is a growing focus on domain-specific applications. Prompt designers are tailoring their approaches and techniques to specific industries and use cases. Domain-specific prompt engineering allows for more accurate and contextually relevant responses, catering to the unique requirements of different domains, such as healthcare, finance, legal, and e-commerce.
Improved User Interfaces: User interfaces for prompt engineering tools are evolving to provide a more intuitive and user-friendly experience. Efforts are being made to simplify the process of designing prompts, configuring AI models, and obtaining desired outputs. Improved user interfaces enable a broader range of users, including non-technical individuals, to engage in prompt engineering and leverage AI technologies effectively.
Data Efficiency and Sample Efficiency: Researchers and practitioners are exploring techniques to improve the data efficiency and sample efficiency of prompt engineering. This involves optimizing the utilization of available data and samples to achieve better performance and reduce the reliance on large-scale datasets. Strategies such as few-shot learning, transfer learning, and data augmentation are being employed to enhance the efficiency of prompt engineering processes.
Challenges and Considerations in Prompt Engineering
While the future of prompt engineering holds great promise, it also presents specific challenges and considerations that need to be addressed:
Bias Mitigation: Bias in AI systems remains a critical concern. Prompt engineering must prioritise the mitigation of biases, both in the training data and the prompts themselves. Robust evaluation frameworks and ongoing research are crucial in ensuring fairness and preventing the amplification of existing biases.
Explainability and Transparency: As AI models become more sophisticated, the need for explainability and transparency becomes increasingly important. Prompt engineering should focus on providing mechanisms for users to understand and interpret the decision-making processes of AI models. Explainable AI techniques, interpretability tools, and comprehensive documentation can contribute to building trust and accountability.
Data Privacy and Security: Prompt engineering often relies on large datasets, raising concerns about data privacy and security. It is vital to handle data responsibly, obtain proper consent, and implement robust security measures to protect user information and prevent unauthorised access.
Scalability and Generalization: Prompt engineering techniques may face challenges in scaling up to handle large-scale datasets or diverse domains. Ensuring that prompt engineering approaches can generalize well across different contexts and applications is crucial for their widespread adoption and effectiveness.
Domain Knowledge and Expertise: Designing effective prompts often requires domain-specific knowledge and expertise. Prompt engineers need to have a deep understanding of the target domain to craft prompts that elicit relevant and accurate responses. Acquiring and maintaining domain expertise can pose a challenge, particularly in rapidly evolving industries or niche fields.
User Experience and Usability: Prompt engineering tools and interfaces should be designed with the user experience in mind. They should be intuitive, user-friendly, and accessible to a wide range of users, including those with limited technical skills. Improving the usability and accessibility of prompt engineering platforms can enhance adoption and empower a broader user base.
Model Robustness and Safety: Prompt engineering must consider the robustness and safety of AI models. Prompt designers should be aware of potential vulnerabilities, adversarial attacks, and unintended consequences that may arise from prompt manipulation. Implementing robustness measures, safety guidelines, and model monitoring mechanisms are essential to mitigate risks.
Ethical and Legal Compliance: Prompt engineering must adhere to ethical standards and legal regulations. Prompt designers should ensure compliance with privacy laws, data protection regulations, and ethical guidelines for AI development and deployment. Ethical considerations, including informed consent, responsible data usage, and preventing harm, should be central to prompt engineering practices.
Exciting Possibilities and Future Applications
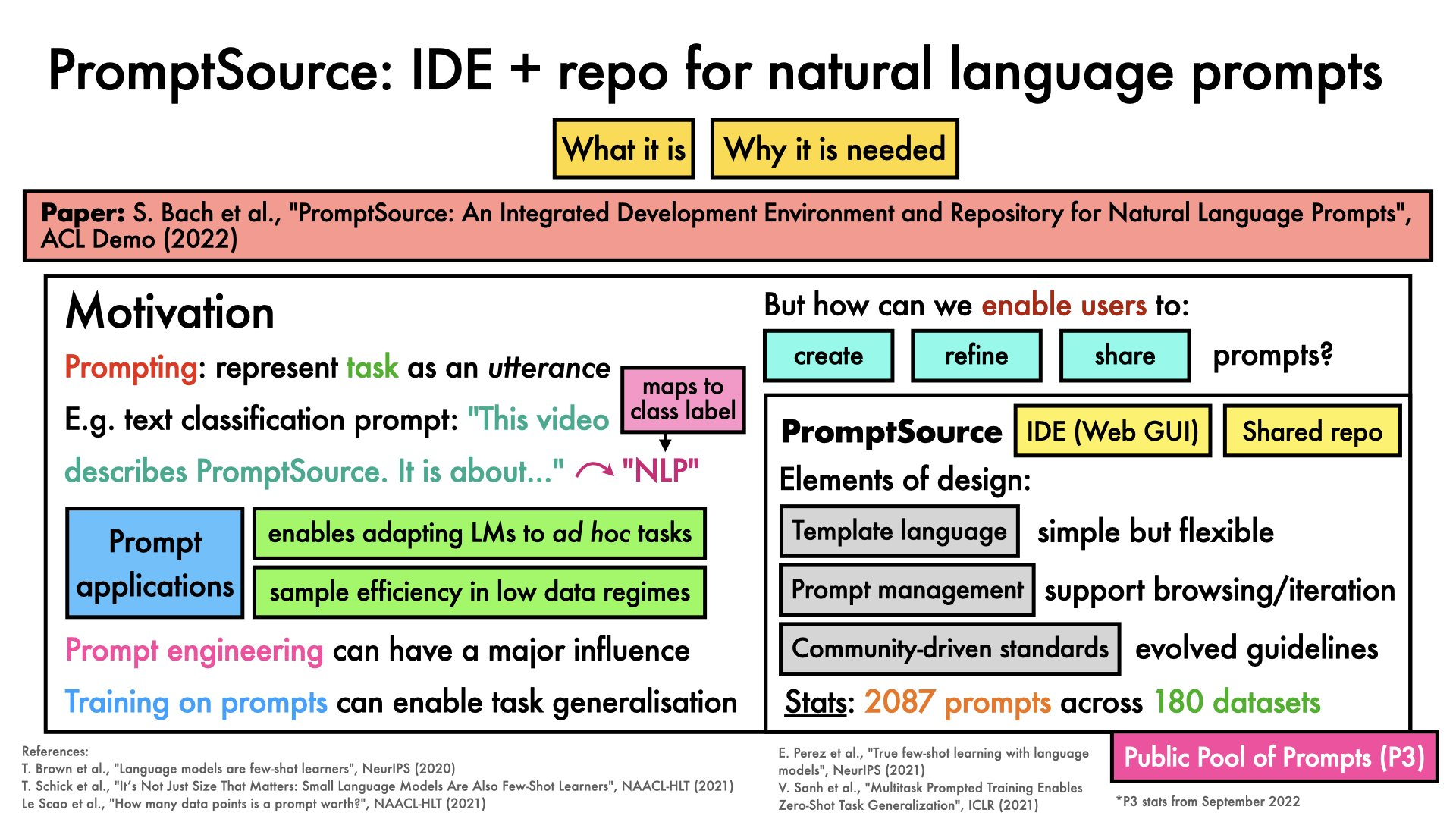
The future of prompt engineering is filled with exciting possibilities. Here are some potential applications and areas where prompt engineering can make a significant impact:
Personalized Healthcare: Prompt engineering can enable AI models to provide personalised healthcare recommendations, assist in remote patient monitoring, and facilitate early disease detection. This personalised approach has the potential to revolutionise healthcare, leading to improved patient outcomes and more efficient healthcare delivery.
Creative Collaboration: Prompt engineering can foster creative collaboration between humans and AI. By generating prompts that inspire and spark creativity, AI models can assist artists, writers, and innovators in their creative processes, unlocking new levels of imagination and innovation.
Decision Support Systems: Prompt engineering can enhance decision support systems by enabling AI models to provide context-aware recommendations and analysis. This can assist professionals in diverse fields such as finance, marketing, and logistics, leading to more informed and data-driven decision-making.
In a Nutshell
Throughout this three-part series, we have learned a lot about prompt engineering. We have explored its fundamental principles, advanced techniques, real-world applications, and emerging trends. From understanding the importance of prompt design to harnessing the power of contextual prompts, multi-modal inputs, and reinforcement learning, we have witnessed how prompt engineering can unlock the full potential of AI models.
In Part 1, we laid the foundation by diving into the basics of prompt engineering, its significance in shaping AI model behavior, and the ethical considerations involved. We learned how prompt engineering empowers users to tailor AI responses and achieve desired outcomes.
Part 2 took us on a deeper exploration of advanced techniques, real-world examples, and case studies. We witnessed the transformative impact of contextual prompts, multi-modal inputs, and reinforcement learning on AI models. From revolutionizing healthcare and education to empowering content creators and enhancing language translation, prompt engineering showcased its versatility and application across diverse domains.
In Part 3, we dived into emerging trends that are shaping the future of prompt engineering. Adaptive prompting, OpenAI's prompting tools, and collaboration within prompt engineering communities highlight the evolving landscape of this field. We also acknowledged the challenges and considerations surrounding bias mitigation, explainability, data privacy, scalability, domain expertise, user experience, model robustness, and ethical compliance.
In conclusion, prompt engineering is a powerful tool that empowers users to shape the behavior of AI models, enhance accuracy, and achieve tailored outcomes.
How much is a great User Experience worth to you?
Browsee helps you understand your user's behaviour on your site. It's the next best thing to talking to them.
